Data-Efficient Sensor Fusion
The goal of data fusion is to optimally combine data from multiple sensors to achieve improved performance for different inference tasks over what could be accomplished by the use of a single sensor. Fusion of multi-modal data acquired at multiple sensors has attracted much attention by the global research community due to its significance in many potential applications including sensor networks designed for environmental monitoring, automated target recognition, remote sensing, battlefield surveillance, industrial, manufacturing, and bio-medical applications; smart camera networks for safety and security applications; cognitive radio networks to address spectrum scarcity; and radar applications.
The goal of this Sensor Fusion project was to develop efficient fusion techniques with high dimensional data for different inference tasks exploiting low dimensional sampling schemes. Efficient handling of data of large dimensions with limited processing and communication capability at individual sensor nodes is a key requirement in multi-sensor data fusion. Reduced dimensional data acquisition techniques address such challenges. Using compressive sensing (CS), a small number of measurements obtained via random projections is sufficient to reliably reconstruct a sparse signal given certain conditions of the projection matrix. Complete signal reconstruction is not necessary for detection, classification, localization and tracking in sensor and radar networks. This project developed efficient fusion techniques for detection and localization tasks when the observations at multiple sensors (in general with different modalities) are compressed via low dimensional random projections. Simulation and experimental data were used to illustrate the effectiveness of the assumed models and developed algorithms for detection and localization.
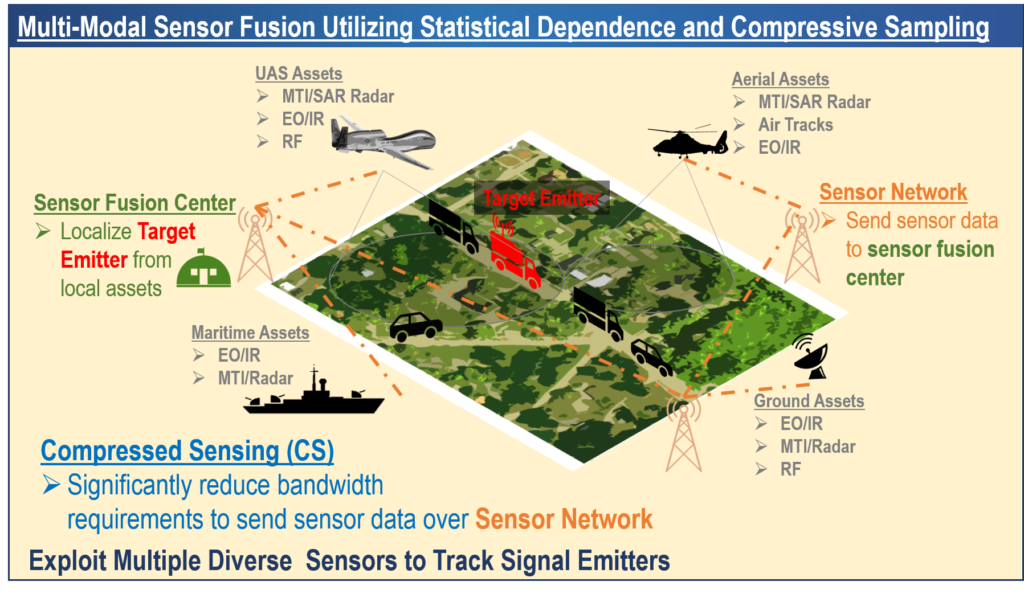
Sensor Resource Management
ANDRO developed a Sensor Resource Management (SRM) solution with the intended mission to help manage heterogeneous sensors in a ballistic missile scenario. Our multi-level management approach is also applicable to any sensor tasking problem where long timelines make it difficult to implement standard scheduling algorithms. We implement a SRM system that optimally assigns one of the several heterogeneous sensors to each task in the kill chain for a multiple missile attack. Our multilevel SRM system is based on stochastic optimization via Markov Decision Processes (MDP). The SRM consists of Local Resource Managers (LRM) which optimize the tasking of individual sensors, and a Global Resource Manager (GRM) which controls system level performance. This MDP approach was previously extensively tested in a laboratory environment for multi-sensor SRM applications.
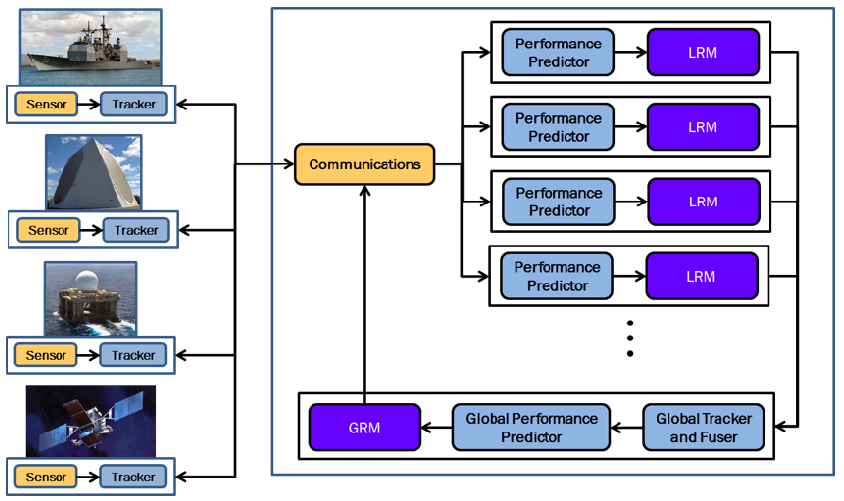
Automated Target Characterization
ANDRO developed and implemented a set of algorithms within a unified target characterization and correlation framework capable of operating in a multiple heterogeneous sensor environment where detection, classification, localization and track priority information is exchanged among multiple platforms. Our goal was to deliver an effective automated and autonomous information extraction and fusion system that can be incorporated in today’s operational systems. The primary focus was on the development of algorithms for target characterization and correlation that can handle the difficult track handover between EO/IR boost phase detection sensors and weapon control sensors.
Information fusion with heterogeneous sensors is challenging because non-kinematic features are different for each sensor type making it is difficult to correlate features across sensors. Thus, it is necessary to develop meta-features that are sensor invariant and amenable to optimal track correlation across sensors. Once this target characterization is carried out effectively, the next task is to develop efficient fusion or correlation algorithms that can yield better common tracks and facilitate accurate track hand-over. In our approach, target characterization (information extraction) and correlation (information fusion) are tightly coupled problems that are addressed jointly to ensure optimal overall performance.
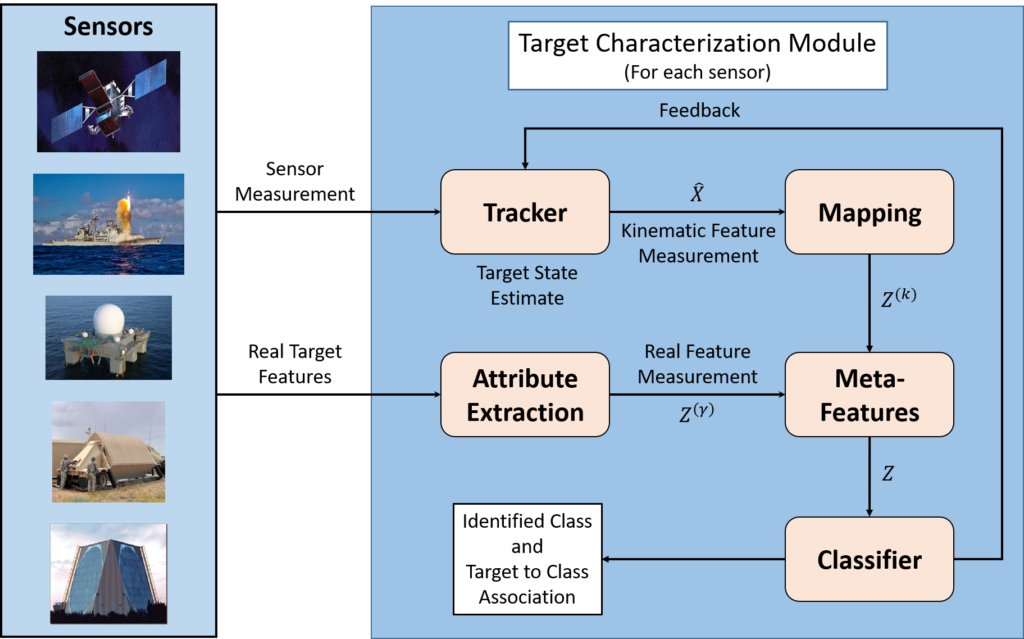
Radar Data Fusion & Registration
The successful DataFusR phase II SBIR project developed processes for fusing information from multiple, widely separated radars together with EO/IR sensor data to significantly enhance the accuracy and reliability of ballistic missile defense (BMD) systems over traditional single radar systems. DataFusR addressed the discrimination and tracking component of the BMD problem by autonomously fusing estimates of the properties for each perceived entity in the threat cloud environment. The DataFusR project demonstrated capabilities to discriminate targets from decoys and clutter, track the targets and display the results in a Single Integrated Air Picture (SIAP). A proposed Multi-Source Data Fusion (MSDF) system could provide intelligent control of DataFusR processes.
This effort both developed new processes and enhanced existing technology necessary to process, and fuse information from multiple radars (either at the same or different frequency) to form a single integrated air picture (SIAP) of the ground midcourse ballistic target environment. DataFusR demonstrated the technology in increasingly more realistic scenarios using simulated data from multiple radars and other multi-modality sensors. DataFusR operated on a combination of sensor measurements, features, track states, and object types to produce a highly accurate SIAP.
DataFusR simulations incorporated theoretical/mathematical target and clutter models derived from the physics of radar. An extensive set of simulation scenarios were generated including single and multiple targets with and without clutter. Both 2D and 3D simulations were performed. The simulations evaluated the performance of the candidate processes utilizing single radars, multiple co-located radars and widely separated radars. The amplitude of the radar returns from frequency diverse sensors was exploited to aid in target/clutter discrimination as well as data association between sensors. Finally data from one or more passive sensors were fused with multiple radar data to further enhance the system performance. An extension to the 3D tracking research involved the development of processes for optimizing the location of the radar systems.
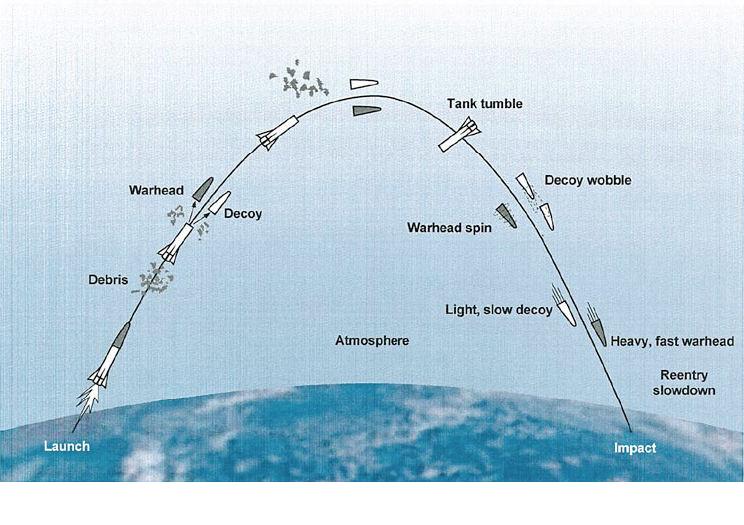
MIMO Radar
The Multiple Input Multiple Output Sensor Acquisition (MIMOSA) project applied MIMO technologies, originally designed for radio communications applications, to the ballistic missile defense challenge in ever increasingly realistic scenarios. In MIMOSA Phase I the MIMO concept was successfully demonstrated for detecting and tracking a single target in two dimensions using spatial diversity. In Phase II the algorithms was extended to target detection and tracking in three dimensions. Comparative analysis was performed for non-coherent, semi-coherent and coherent MIMO radar systems. Algorithms were adapted for detecting and tracking of multiple targets.
The MIMOSA objective is to collect, process, and fuse information in real time from multiple radars that are widely distributed spatially (spatial diversity) and which operate with different frequencies or waveforms (frequency and waveform diversity). This synergistic MIMO-based approach promises to provide a more accurate picture of the adversary threat cloud than any single sensor or group of co-located sensors operating independently can offer.
The MIMO methodology was enhanced to include Doppler estimation and feature extraction for radars operating with different frequencies and waveforms. The goal of the MIMOSA project was to demonstrate three dimensional feature-aided detection and tracking of multiple targets in the presence of decoys and clutter.
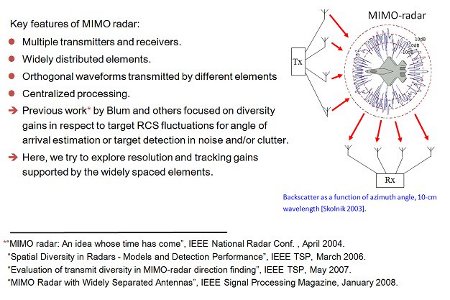