ANDRO Marconi-Rosenblatt Artificial Intelligence Innovation Lab
In 2019 ANDRO launched the Marconi-Rosenblatt Artificial Intelligence Innovation Lab, named in honor of Guglielmo Marconi and Frank Rosenblatt, two pioneers notable in the fields of radio communications and artificial intelligence, respectively. The lab will focus on applying artificial intelligence solutions for next-generation wireless networking to advance the state of the art in Internet-of-Things technologies, space communications and other customer applications. The pioneering solutions will also be applied to natural language processing, speech recognition, computer vision, big-data analytics, brain-computer interfaces and intelligent solutions for a better AI-enabled future. The lab’s applied research will directly impact healthcare, education, transportation, security, smart cities, and defense markets among others.
What do we do?
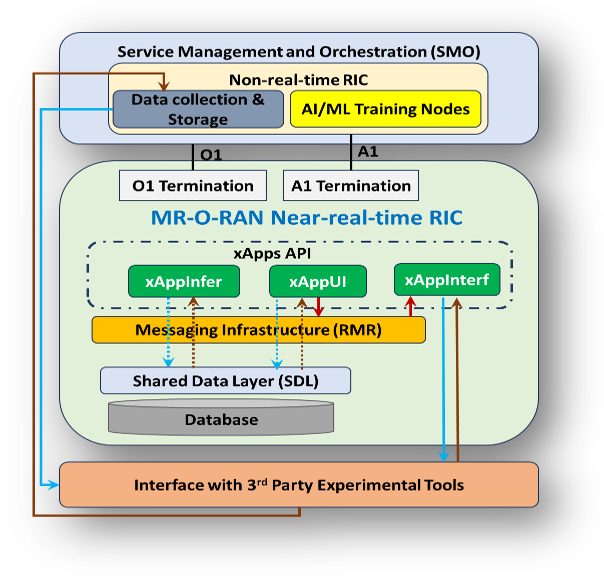
Beyond 5G
UAV, AI, and Zero Trust Inspired Next-generation Network Optimization
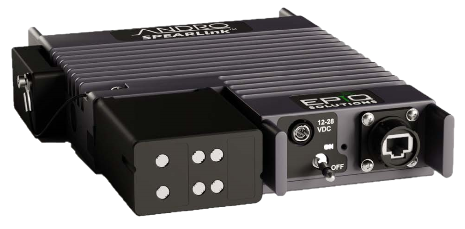
Intelligent Mesh Networking
Reinforcement learning-based mesh networking for terrestrial, aerial, and space networks
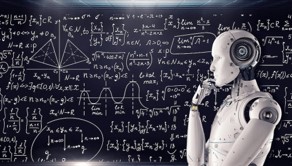
Safe AI
Approaches for online planning problem to utilize safety and utility simultaneously
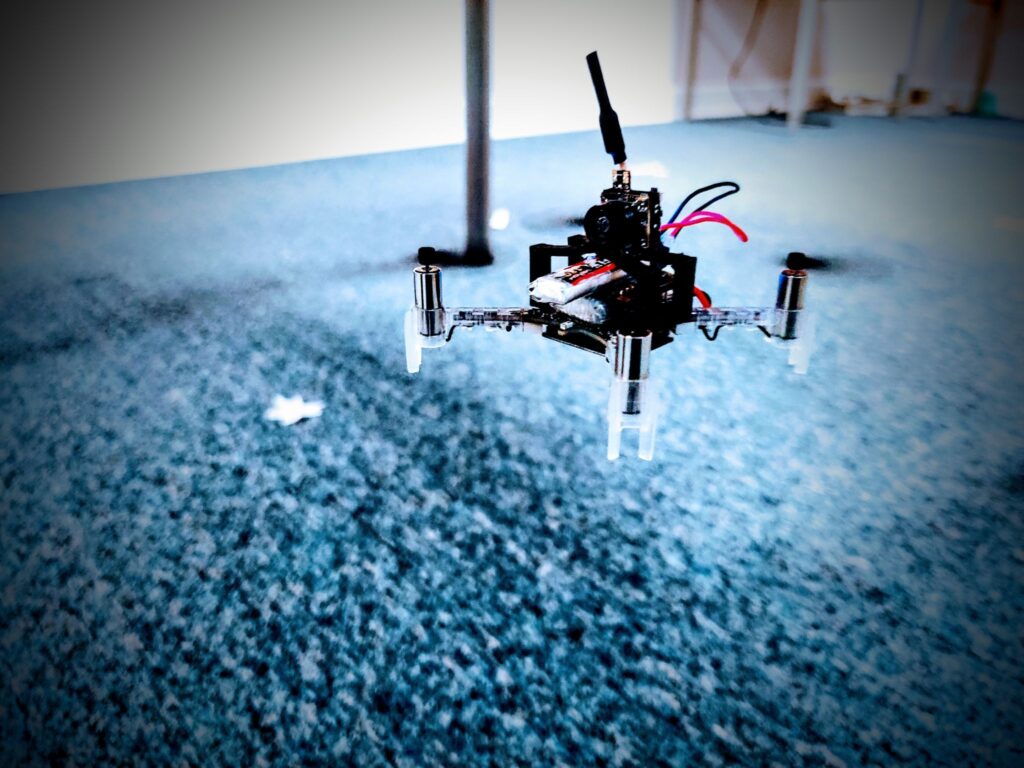
Autonomous UAVs
Artificial neural network and reinforcement learning enabling autonomous flight control for UAVs
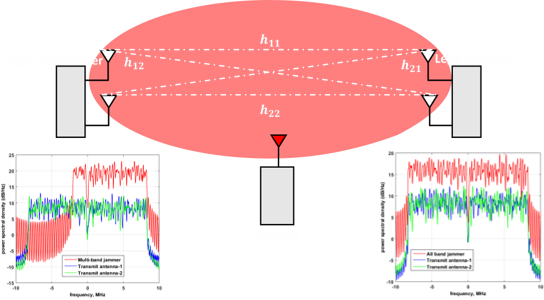
Jammer Resilient Communication
MIMO-beamforming techniques along with hybrid adaptive link layer for Jammer-resilience
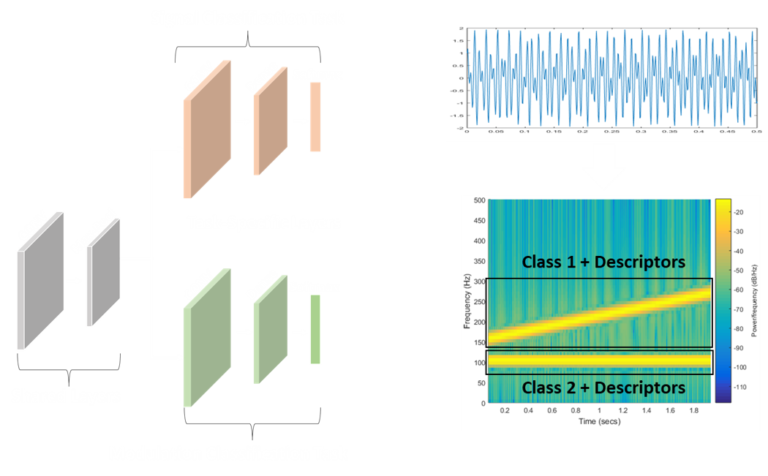
RF Signal Intelligence
Multi-task learning framework for radio frequency signal classification and characterization
Latest AI News
-
Dr. Jithin Jagannath – Panelist at 2023 AUSA Annual Meeting & Exposition
Dr. Jithin Jagannath, Founding Director of ANDRO's Marconi-Rosenblatt AI Innovation Lab has been invited to participate in a Panel titled, "Army Applied Small Business Innovation Research (SBIR) and xTech Panel" moderated by Dr. Matt Wills, Director, Army Prize Competitions. He will be discussing his experience developing Radio Frequency Machine Learning (RFML) solutions for spectrum awareness and how the Army CATALYST Award will help accelerate its maturation. Please Join them next week on Tuesday, 10th Oct at 1500-1615 at AUSA Annual Meeting & Exposition. ANDRO will also have a Booth on 11th Oct that showcases several of our technologies. Visit our team there to learn more. Learn More About AUSA Here: https://meetings.ausa.org/annual/2023/ -
ANDRO Announced as U.S. Army AI/ML Applied SBIR Program Awardee
ANDRO Computational Solutions, LLC and its Marconi Rosenblatt AI/ML Innovation Lab is proud to announce its recent SBIR award through the Army Applied SBIR Program in its first AI/ML-focused open-topic solicitation. The U.S. Army’s data-intelligence capabilities require novel artificial intelligence and machine learning technologies to ensure the American soldier can overcome any adversary on any battlefield, real world or digital. In the AI/ML-focused open-topic solicitation, the Army Applied SBIR Program awarded 39 small-business contracts to deliver AI/ML solutions — ranging from natural language processing to data analysis and labeling — to identify information such as images, spoken words, text files and videos to build out machine-learning models. These models use the data to “teach” AI and improve the accuracy of information within their database. “Through this open-topic solicitation, the program received a record-setting 420 proposals for disruptive technologies that inject game-changing innovation into Army programs,” said Dr. Matt Willis, director of Army Prize Competitions and the Army Applied SBIR Program. “Additionally, these innovations tackle critical modernization challenges while advancing the goals of the National Defense Strategy.” The companies each received an Army Small Business Innovation Research Phase I contract up to $150,000 to develop AI/ML solutions. Utilizing the Army’s investment, the businesses will... -
IEEE Communications Society’s Flagship Conference Presentation
Anu Jagannath, Associate Director of ANDRO's Marconi-Rosenblatt AI/ML Innovation Lab is presenting at IEEE Communications Society's Flagship conference IEEE Global Communications Conference (GLOBECOM) on Tuesday, December 6, 2022. She will be discussing an innovative approach for RF Fingerprinting in the recent paper "RF Fingerprinting Needs Attention: Multi-task Approach for Real-World WiFi and Bluetooth". Read the Full Paper Here IEEE Global Communications Conference (GLOBECOM) is one of the IEEE Communications Society’s two flagship conferences dedicated to driving innovation in nearly every aspect of communications. Each year, more than 3000 scientific researchers and their management submit proposals for program sessions to be held at the annual conference. After extensive peer review, the best of the proposals are selected for the conference program, which includes technical papers, tutorials, workshops and industry sessions designed specifically to advance technologies, systems and infrastructure that are continuing to reshape the world and provide all users with access to an unprecedented spectrum of high-speed, seamless and cost-effective global telecommunications services. The 2022 IEEE Global Communications Conference (GLOBECOM) will be held in the warm and wonderful city of Rio de Janeiro, Brazil, from 4 to 8 December 2022. Themed “Accelerating the Digital Transformation through Smart Communications,” this flagship conference of the IEEE Communications Society... -
ANDRO Presents at the 2022 International Conference on Machine Learning
We are looking forward to the International Conference on Machine Learning, 2022 #ICML where Nicholas Polosky, the lead author will be presenting our work on Wednesday (20th). Please join him if you are planning to participate this year. As many of you are aware, an area of expertise in our MR Lab is applying machine learning for vision-based UAV autonomy. We have been developing some of these capabilities for our Naval Air Systems Command (NAVAIR) customer. In such cases, when the risk associated with the agents learning online is high, we have to resort to offline learning with an emphasis on applying safety-related constraints. In such deployment scenarios, a robust guarantee on the cost of a policy is paramount in real-world scenarios where breaking a cost budget may carry extreme consequences. To address some of the associated challenges, in this paper, we introduce Constrained Offline Policy Optimization (COPO). N. Polosky, BC. da Silva, M. Fiterau, J. Jagannath, "Constrained Offline Policy Optimization", in Proc. of the 39th International Conference on Machine Learning (ICML), Baltimore, MD, July 2022 Reach out of you are interested in learning more about our #autonomy solutions for #unmanned systems! For more information, visit: Getting Started (icml.cc)
AI for Wireless
Select Publications
A. Jagannath, Z. Kane, J. Jagannath, “RF Fingerprinting Needs Attention: Multi-task Approach for Real-World WiFi and Bluetooth” in Proc. of IEEE Global Communications Conference (GLOBECOM), Rio de Janeiro, Brazil, December 2022.
A. Jagannath, J.Jagannath, T. Melodia, ”Redefining Wireless Communication for 6G: Signal Processing Meets Deep Learning with Deep Unfolding”, IEEE Transactions on Artificial Intelligence, vol. 2, no. 6, pp. 528-536, Dec. 2021. [Download]
J. Jagannath, K. Hamedani, C. Farquhar, K. Ramezanpour, A. Jagannath, “MR-iNet Gym: Framework for Edge Deployment of Deep Reinforcement Learning on Embedded Software Defined Radio”, in Proc. of ACM Workshop on Wireless Security and Machine Learning (WiseML), San Antonio, Texas, USA May, 2022. [Best Paper Award] [Download]
A. Jagannath, J. Jagannath, “Multi-task Learning Approach for Modulation and Wireless Signal Classification for 5G and Beyond: Edge Deployment via Model Compression”, Physical Communications (Elsevier), vol. 54, 101793, ISSN 1874-4907, 2022. [Download]
J. Jagannath, K. Ramezanpour, A. Jagannath, “Digital Twin Virtualization with Machine Learning for IoT and Beyond 5G Networks: Research Directions for Security and Optimal Control”, in Proc. of ACM Workshop on Wireless Security and Machine Learning (WiseML), San Antonio, Texas, USA May, 2022. [Download]
Select Projects
DeepSPEC®
Efficient Multi-task Machine Learning for Signal type and Modulation Classification for Edge Deployment
Tactical systems are often presented with an amalgam of RF signals emanating from friendly, hostile and neutral sources. Blind signal identification techniques that can identify RF signals without any prior knowledge can play a significant role in enhancing the spectrum visualization. Such blind signal identification techniques would need large datasets to learn the inherent signal characteristics to allow a SIGINT system to accurately classify the signal. To surpass this hurdle, we use the powerful AI tool, “Deep Convolutional Neural Network (DCNN)” to extensively train, test and validate on large RF datasets. The SIGINT module are usually mounted as an adjunct to the tactical systems or as a portable device that can be carried by the operators or mounted on their vest and therefore, it is ideal for them to be of small form-fit, efficient in power consumption, and offer real-time performance. DeepSPEC® offers:
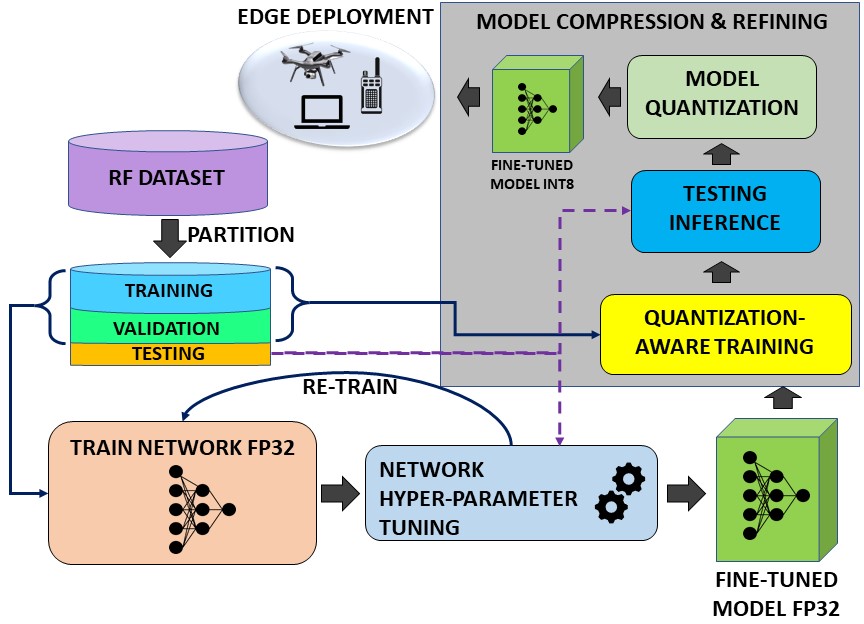
-
Power Efficient Hardware Acceleration
The widely used GPU accelerators consume large amount of power, therefore, their operation in low SWaP devices becomes challenging. On the other hand, FPGA based accelerators are gaining momentum for low SWaP platforms due to their higher power efficiency (i.e., performance per Watt), parallelism for real-time performance, reconfigurability, and low development cost. Ensuring the performance on FPGA relies on algorithmic optimization techniques such as bit width optimization, selective network pruning, weight quantization, loop-level parallelism, memory access, among others.
-
Diverse RF Signal Types
An advanced SIGINT system must be able to handle and identify a vast and diverse class of tactical LPI/LPD (soldier radio waveforms, wideband networking waveforms, among others), general communication, and jammer signals to present a clear spectrum situational awareness. Such a rich and diverse RF environment will require a powerful tool such as that offered by AI to identify the myriad waveforms under a range of operational scenarios.
-
Advanced, Extensible, Blind Signal Analysis
Above all, the SIGINT system will have no prior knowledge of the RF signals under consideration. With increasing advancements in signal processing, the tactical systems continue to adopt enhanced waveforms to strive in the challenging operational scenarios. Thus, a SIGINT system for the evolving combat scenarios must be able to accommodate new RF signal types, perform blind signal identification and analysis to portray the hidden features of the incident RF signal.
-
COTS-based Open Architecture
The RF waveforms of tactical systems are continuously evolving, and an inflexible architecture will soon become outdated due to the inability to handle modern signal types. Therefore, a SIGINT device must be reconfigurable to allow affordable future waveform inclusion with ease and possess an open architecture to allow seamless integration to legacy systems.
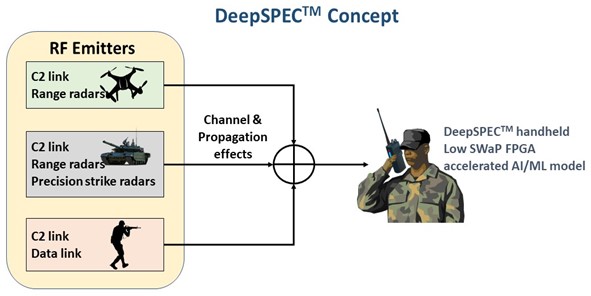
The proof-of-concept of DeepSPEC® was established and following are the key accomplishments:
- A comprehensive dataset of ground-based radar, aerial radar, and communication signals were generated in simulation and with actual radios. Simulations considered vast dynamic hardware and propagation effects those that cannot be mimicked over actual radios to ensure robutst operation of DeepSPEC® under challenging conditions.
- DeepSPEC® was able to perform both modulation and signal classification with 98% accuracy on the simulation dataset and 99% accuracy on the radio generated dataset.
- DeepSPEC® offered short-time Fourier transform and Wigner-Ville spectrograms to enable enhanced waveform visualization for operators. A supporting GUI for waveform visualization was developed in C++.
- DeepSPEC® performance was contrasted with 8 current state-of-the-art schemes to demonstrate superior classification performance.
- DeepSPEC® model was compressed to 11.8x reduced size with 99% modulation and signal classification accuracies.
- DeepSPEC® SIGINT was identified to offer low SwaP.
DeepeRFind
RF Fingerprinting Approach for Commercial IoT Devices
Situational awareness and understanding the battlespace is a fundamental enabler across all tactical systems. To this end, DeepeRFind is being designed to be a small form-fit, multi-task Machine Learning (ML) based fingerprinting device. DeepeRFind: Sensitivity Enhancing Radio Frequency Fingerprinting Technique using Deep Learning – which performs a comprehensive RF fingerprinting scheme with emphasis on enhancing receiver sensitivity. DeepeRFind is envisioned to – mitigate adversarial spoofing/masquerading, enable confidential communication, enhanced situation awareness by extracting the hardware specific signatures enabling emitter recognition by low SWaP-C embedded platforms.
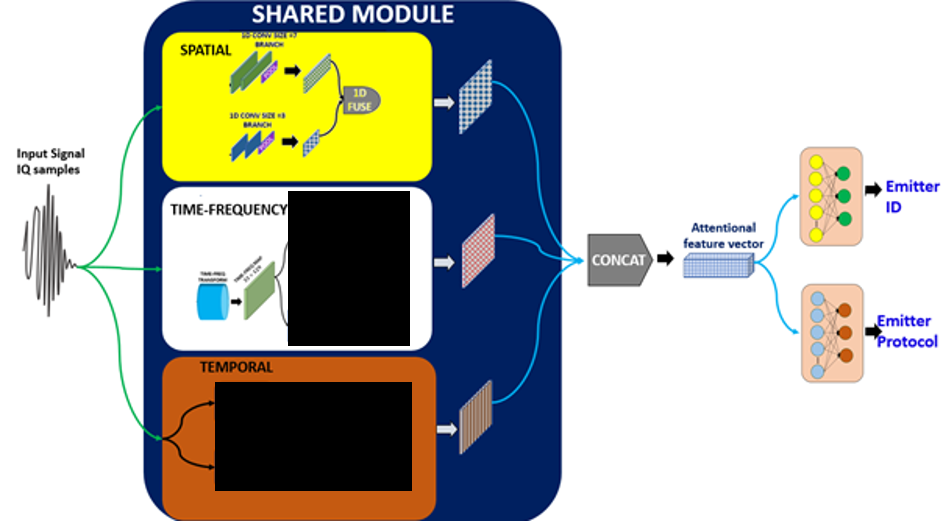
DeepForecaster
Comprehensive SIGINT for RF Signal Detection, 3D Localization, and Behaviors Prediction
DeepForecaster is being developed to be a comprehensive SIGINT unit that performs multiple SIGINT tasks under one hood. The all-in-one functionality is achieved with superior AI engine that processes raw IQ samples from the radio frontend. DeepForecaster is envisioned to provide precise PNT, signal identification/classification, emitter tracking, and radio environment modeling under a severe dynamic EM environment. Another main task of DeepForecaster is to self-learn to predict the spectrum behavior to provide actionable information regarding upcoming spectrum usage. DeepForecaster will be designed to operate seamlessly and track/geolocate the interference sources in a GPS denied environment – rendering it ideal for tactical setting.
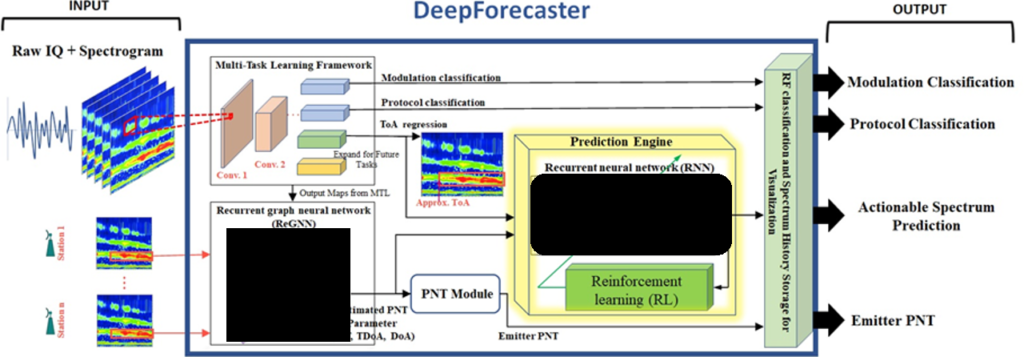
DeepLink®
Reinforcement learning for real-time wireless resource allocation
Embedded Machine Learning for autonomous SDR transmission parameter control based on situational awareness.
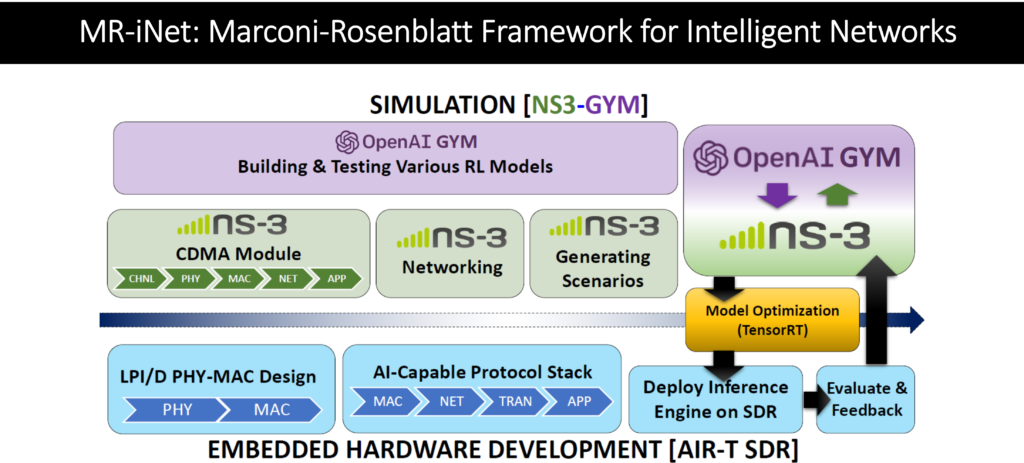
5G and Beyond
Select Publications
K. Ramezanpour, J. Jagannath, “Intelligent Zero Trust Architecture for 5G/6G Networks: Principles, Challenges, and the Role of Machine Learning in the context of O-RAN”, Computer Networks (Elsevier), vol. 217, 109358, ISSN 1389-1286, 2022.
A. Jagannath, J. Jagannath, “Multi-task Learning Approach for Modulation and Wireless Signal Classification for 5G and Beyond: Edge Deployment via Model Compression”, Physical Communications (Elsevier), vol. 54, 101793, ISSN 1874-4907, 2022. [Download]
K. Ramezanpour, J. Jagannath, A. Jagannath, “Security and Privacy vulnerabilities of 5G/6G and WiFi 6: Survey and Research Directions from a Coexistence Perspective”, Computer Networks (Elsevier), arXiv preprint arXiv:2206.14997, 2022.
J. Jagannath, K. Ramezanpour, A. Jagannath, “Digital Twin Virtualization with Machine Learning for IoT and Beyond 5G Networks: Research Directions for Security and Optimal Control”, in Proc. of ACM Workshop on Wireless Security and Machine Learning (WiseML), San Antonio, Texas, USA, May, 2022. [Download]
Select Projects
Wi5G
Secure Spectrum Coexistence Technology for 5G and WiFi6
Project Wi5G aims to design novel technology to sustain secure, fair, collaborative, spectrum efficient WiFi 6/6E and 5G cellular coexistence. Wi5G is a software abstracted secure RAN orchestrator that leverages the 3GPP standardization features to enable harmonious coexistence with WiFi networks. Wi5G is primarily designed to enable interoperability and accelerated adoption capabilities by legacy systems as well as standalone 5G architectures. Wi5G ensures secure and tokenized spectrum sharing to ensure subscriber privacy and prevent unauthorized user access. Wi5G is a realization of cognitive capability deployment at the network edge. Wi5G foresees immense commercialization potential and estimates a large customer base from the Federal as well as commercial sector for smart city, Industry 4.0, uninterrupted connectivity at public WiFi hotspots, etc.
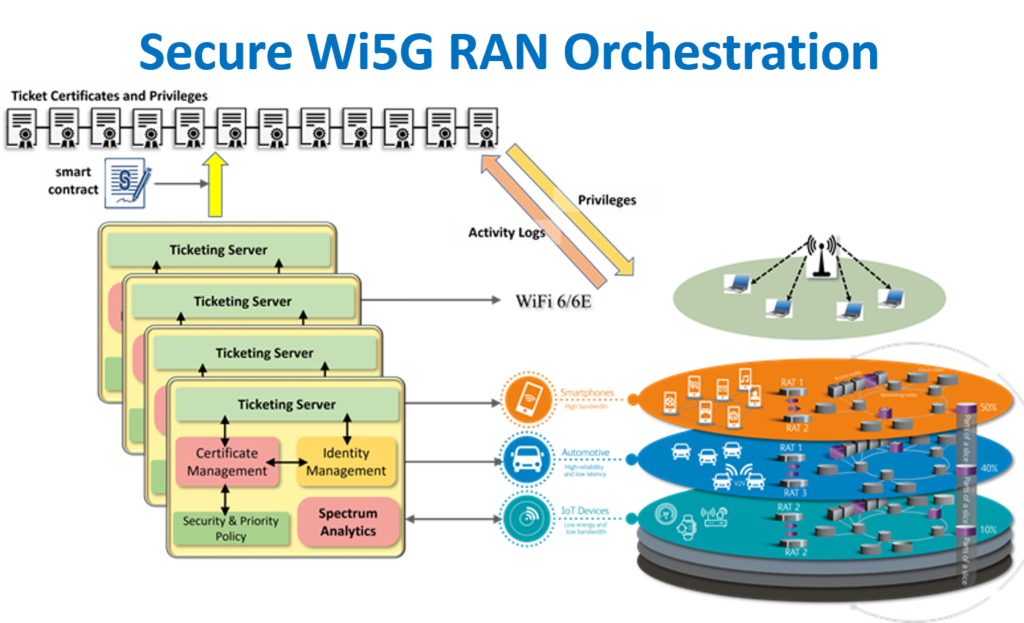
Zero Trust for B5G
Intelligent zero trust architecture for 5G/6G networks
Fifth-generation (5G) wireless networks are expected to handle a large volume of data generated by a wide range of devices including smartphones, autonomous vehicles, smart buildings, cities, and infrastructure. The members of society nowadays have their personal sensitive data stored on servers throughout the network and rely on the various access points to avail network-based services and applications. More importantly, the operation of future critical technologies such as autonomous vehicles, smart grids and emergency systems, that deal with public safety and national security, highly rely on network-based data management and processing. Zero trust architecture (ZTA) is an emerging and necessary framework for cybersecurity to provide the privacy and security of personal and sensitive data in such dynamic environment, which is also introduced by the U.S. Department of Defense (DoD) as the basis for next generation security systems. To the best of our knowledge, this is the first work that discusses how zero trust principles can become the driving force in ensuring security for future wireless networks (5G/6G). In addition, we introduce an O-RAN compliant conceptual intelligent ZTA framework based on artificial intelligence (AI) engines and discuss how the existing AI algorithms can be exploited to realize the premises of a ZTA.
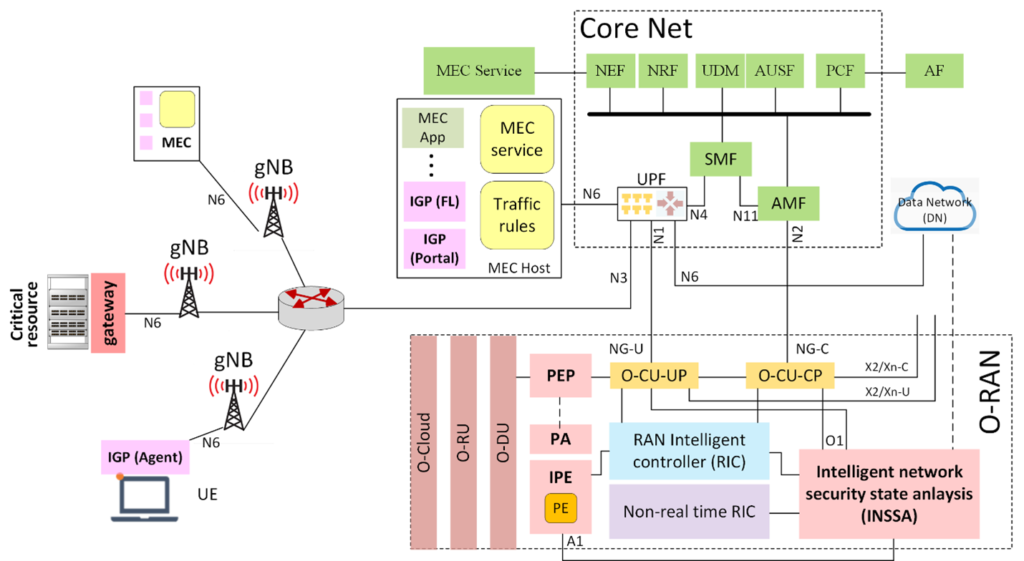
Digital Twin for B5G
Digital Twin Virtualization with Machine Learning for IoT and 5G/6G Networks
Digital twin (DT) technologies have emerged as a solution for realtime data-driven modeling of cyber physical systems (CPS) using the vast amount of data available by Internet of Things (IoT) networks. In this position paper, we elucidate unique characteristics and capabilities of a DT framework that enables realization of such promises as online learning of a physical environment, real-time monitoring of assets, Monte Carlo heuristic search for predictive prevention, on-policy, and off-policy reinforcement learning in real-time. We establish a conceptual layered architecture for a DT framework with decentralized implementation on cloud computing and enabled by artificial intelligence (AI) services for modeling and decision-making processes. The DT framework separates the control functions, deployed as a system of logically centralized process, from the physical devices under control, much like software-defined networking (SDN) in fifth generation (5G) wireless networks. To clarify the significance of DT in lowering the risk of development and deployment of innovative technologies on existing system, we discuss the application of implementing zero trust architecture (ZTA) as a necessary security framework in future data-driven communication networks.
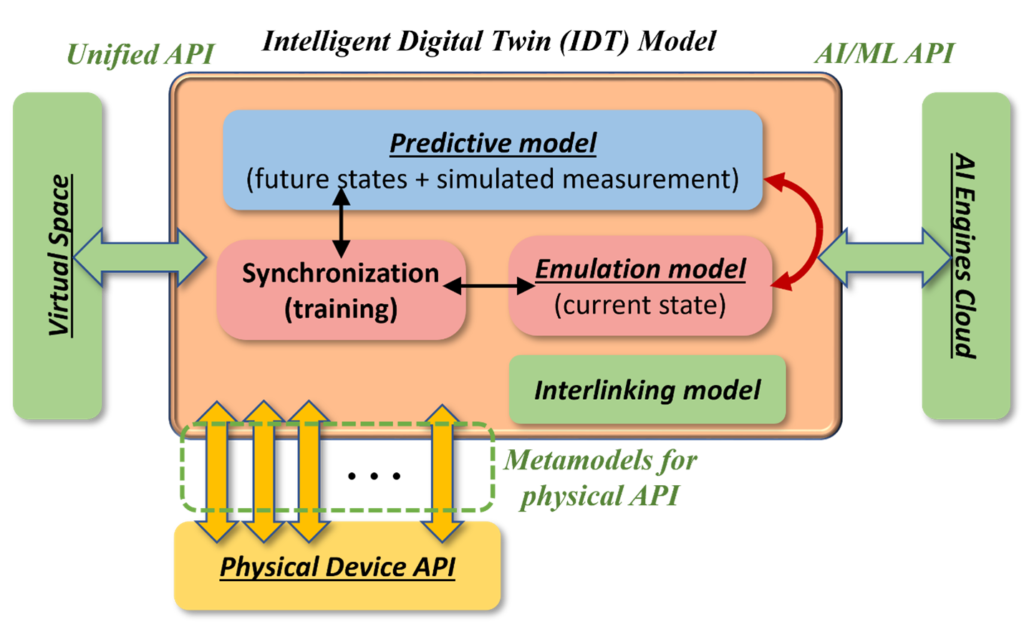
Advanced Signal Processing & Networking
Select Publications
A. Jagannath, J. Jagannath, A. Drozd “High Rate-Reliability Beamformer Design for MIMO-OFDM System under Hostile Jamming”, in Proc. of 29th International Conference on Computer, Communication and Networks (ICCCN), Honolulu, HI, USA, Aug, 2020. [Download]
A. Jagannath, J. Jagannath, A. Drozd, “Towards Higher Spectral Efficiency: Rate-2 Full-Diversity Complex Space-Time Block Codes,” in Proc. of IEEE Global Communications Conference (GLOBECOM), Waikoloa, HI, USA, December 2019. [Download]
J. Jagannath, A. Jagannath, J. Henney, T. Gwin, Z. Kane, N. Biswas, A. Drozd, “Design of Fieldable Cross-Layer Network using Embedded Software Defined Radios: Survey and Novel Architecture with Field Trials”, Computer Networks (Elsevier), vol. 209, 108917, ISSN 1389-1286, 2022. [Download]
J. Jagannath, A. Jagannath, J. Henney, N. Biswas, T. Gwin, Z. Kane, A. Drozd, “Fieldable Cross-Layer Optimized Embedded Software Defined Radio is Finally Here!”, in Proc. of IEEE Conference on Military Communications (MILCOM), San Diego, CA, USA, Nov 2021. [Download]
Select Projects
ARROWLink®
Adaptive Radio for RObotic Warfare (ARROW)
Undeterred wireless communication is the key to every successful mission. From deployed troops to law enforcement officials, lack of reliable communication increases risk and compromises safety. These challenges are further exacerbated when unmanned ground robot vehicles are operated in urban scenarios with severe multipath due to cluster of buildings along with active transmissions from unfriendly jammers. A cognitive communication system must be able to adapt to contested operating environment and mitigate interferences from nearby parallel operating radios, intentional hostile jamming, and multipath fading effects. The SDR communication system can be leveraged to provide agility to the radio in terms of operating frequency, temporal-spatial diversity, transmission rate and power to suit the varying operational requirements and channel conditions. To ensure reliable communication, ANDRO’s MR Lab has designed and developed ARROWLink® with adaptive MIMO-MANET to sustain communications in contested tactical environment.
The proof-of-concept was established with following key accomplishments:
- ARROWLink® can operate at twice the throughput of any other MIMO device that operates using Alamouti or similar rate-1 code in the same operating environment.
- Designed very low complexity STBC decoder.
- Precoding guarantees a lower error rate performance along with significant power savings implying enhanced jammer resilience.
- Demonstrated ARROWLink®’s MIMO-MANET jammer-resilience and sustained link connectivity under unreliable operating conditions.
- Our evaluation results imply in real tactical scenario where the channel conditions can deteriorate dynamically, ARROW’s agile adaptive protocol stack will maintain connectivity delivering acceptable throughput rates while conventional system will suffer link breakage.
- ARROWLink® attains a throughput twice that of conventional in the good channel state and maintains acceptable rate under poor channel conditions.
- ARROWLink® has been evaluated in outdoor over-the-air setting with multiple kinds of jammers.
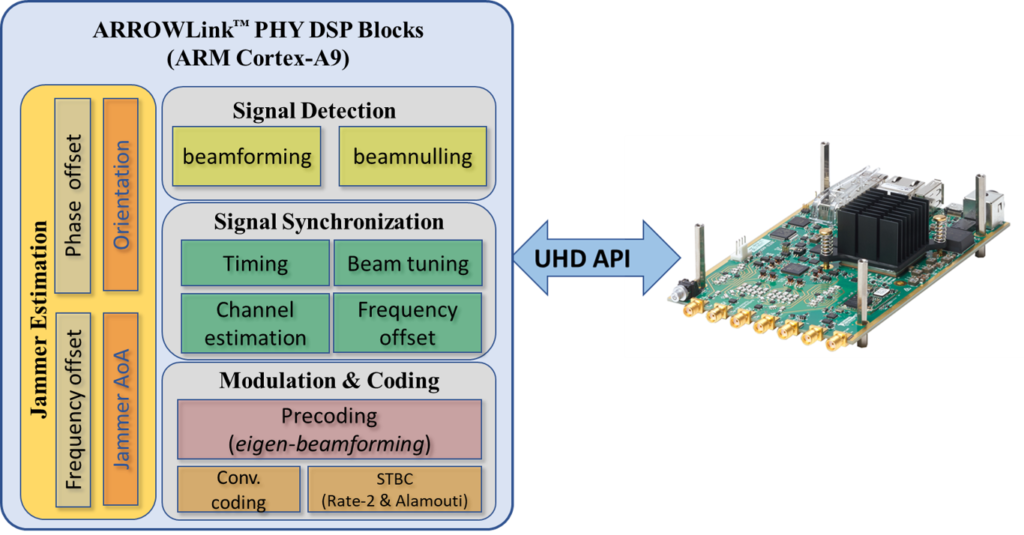
Demonstration of Jammer-resilient LPI/D Waveform
Team has Demonstrated a jammer resilient physical layer with low probability of intercept/detection (LPI/D) properties on an embedded software defined radio (SDR) testbed. The entire LPI/D Direct Sequence Code Division Multiple Access (DS-CDMA) physical layer is executed on ARM Cortex-A57 processor of an SDR. To accomplish this, the physical layer software design adopts a multi-threading architecture with several optimizations in memory accesses to minimize processing time of the receiver and maximize sampling rate. To suppress narrowband jammers, we implement and compare two methods of supervised and semi-supervised auxiliary vector filtering. We demonstrate the LPI/D and jammer resiliency properties of the software-defined DS-CDMA physical layer by real-time streaming of a video in the presence of a strong OFDM signal modeling a narrowband jammer.
SPEARLink®
Developed by ANDRO’s Marconi-Rosenblatt Innovation Lab, SPEARLink® is the first of its kind patented energy-aware mesh networking solution designed using embedded software-defined radio. A scalable solution that allows you to add and remove radios to the network with ease. SPEARLink® mesh network supports AES encrypted information exchange.
Ruggedized and weatherized design built for outdoor deployment. The product comes with a Graphical User Interface (GUI) that provides a single point of remote monitoring and control of the units. It can be used to monitor location, check the health status of the radios, battery levels, and change parameters like data rate, transmission power remotely.
Check out the SPEARLink® Product Page for more information on the hardware and inquiries to purchase SPEARLink®!
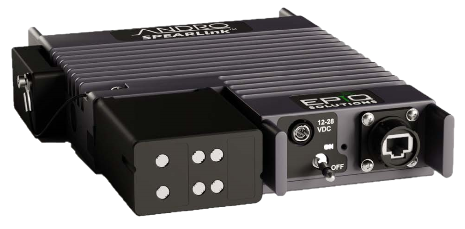
HELPER Network
ANDRO’s Heterogeneous Low PowEr Radio (HELPER) Network is a low cost, low SWaP, intelligent IoT device that is enabling next generation smart city solutions by elevating proprietary energy-aware cross-layer routing protocol.
Check out the HELPER Network dedicated page for more information on HELPER!
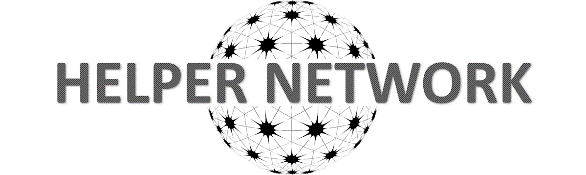
Autonomy for Unmanned Systems
Select Publications
N. Polosky, BC. da Silva, M. Fiterau, J. Jagannath, “Constrained Offline Policy Optimization”, in Proc. of the 39th International Conference on Machine Learning (ICML), Baltimore, MD, July, 2022.
J. Jagannath, A. Jagannath, S. Furman, T. Gwin, “Deep Learning and Reinforcement Learning for Autonomous Unmanned Aerial Systems: Roadmap for Theory to Deployment” in Deep Learning for Unmanned Systems. Studies in Computational Intelligence, Koubaa A., Azar A.T. (eds), vol 984. Springer, Cham., 2021. [Download]
N. Polosky, T. Gwin, S. Furman, P. Barhanpurkar, J. Jagannath, “Machine Learning Subsystem for Autonomous Collision Avoidance on a small UAS with Embedded GPU”, in Proc. IEEE International Workshop on Communication and Networking for Swarms Robotics, 2022. [Download]
Select Projects
RANGER
AI for Enhancing Autonomous Manned Unmanned Teaming (MUM-T)
RANGER is envisioned to be a modular software solution designed to increase mission efficiency and survivability by adapting negation techniques on-the-fly in response to dynamic enemy radar behavior. RANGER agents (manned and unmanned units) are equipped with multi-task learning-based counter-radar intelligence module that provides agents with a common electromagnetic operating picture and an intelligent radar defeat engine that computes both single agent and coordinated defeat methods tailored to the specific threat. Operating in conjunction with the two previous modules is RANGER’s federated learning technique, enabling fast adaptation and behavior change to varying mission conditions, which provides the framework with the requisite battlefield agility to defeat the dynamic radar networks of the future.
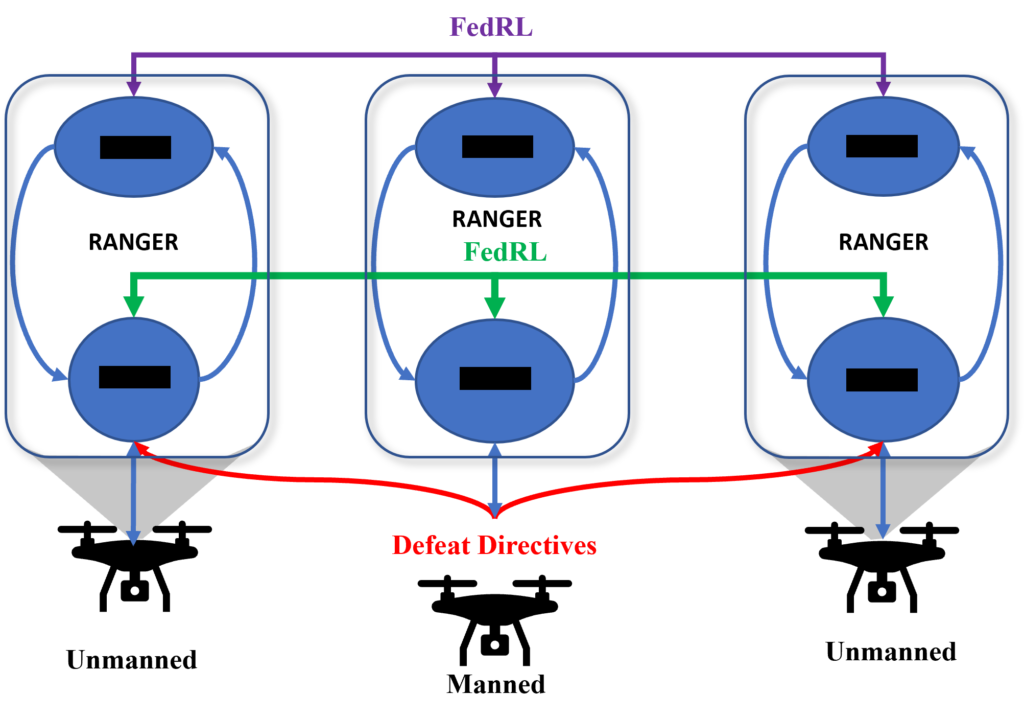
D-MARVEL
Technology to Enhance UAS Autonomy and Collision Avoidance through Machine Vision
D-MARVEL is a novel, general-purpose UAV autonomy framework providing robust perception, collision avoidance, and human-machine teaming for small UAS missions. Importantly, D-MARVEL’s full capabilities are enabled using only basic inertial sensors and a monocular RGB camera. This is key since it enables the UAV to operate using minimal Low SWaP sensors. Such capability is powered by leveraging both machine learning-based and analytic autonomy components whose interaction provides state-of-the-art sensor enhancement and robust algorithmics. D-MARVEL has been successfully flight tested and executed on COTS quadcopters equipped with Low SWaP embedded GPU devices (such as Nvidia Jetson Xavier board) in laboratory settings.
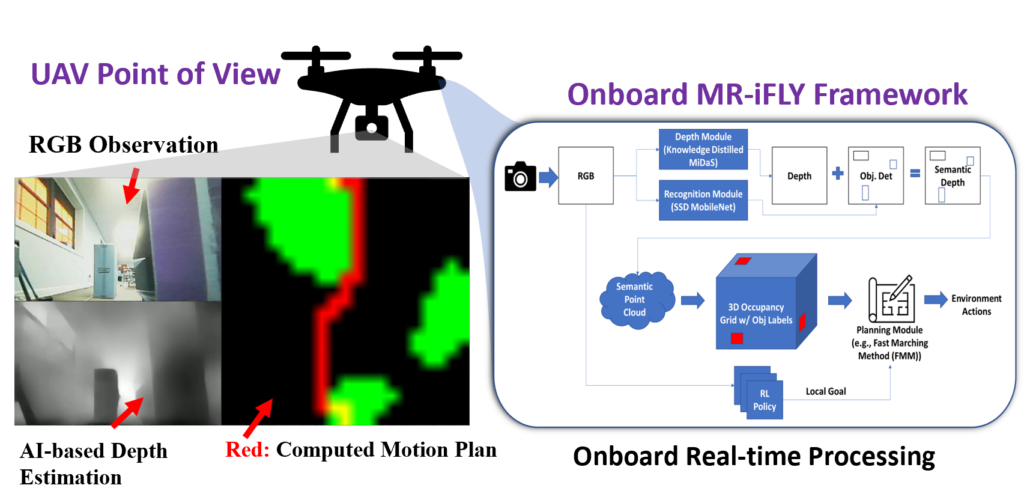
Open-source Code and RF Datasets
ANDRO is committed to contributing to industry-wide open source data, software, and standards. This section will list open-source code and datasets made available to the community by ANDRO’s Marconi-Rosenblatt AI Lab.
Wi-Fi and Bluetooth Dataset for RF Fingerprinting
REAL-WORLD COMMERCIAL WI-FI AND BLUETOOTH DATASET FOR RF FINGERPRINTING
A real-world radio frequency (RF) fingerprinting dataset for commercial off-the-shelf (COTS) Bluetooth and WiFi emitters under challenging testbed setups is presented in this dataset. The chipsets within the devices (2 laptops and 8 commercial chips) are WiFi-Bluetooth combo transceivers. The emissions are captured with a National Instruments Ettus USRP X300 radio outfitted with a UBX160 daughterboard and a VERT2450 antenna. The receiver is tuned to record a 66.67 MHz bandwidth of the spectrum centered at the 2.414 GHz frequency. This is a first-of-its-kind dataset for fingerprinting WiFi-Bluetooth combo chipsets that are commonly found in today’s IoT era. The dataset is split into two for WiFi and Bluetooth – Day1 and Day2 – each of which is recorded in a different time frame but under the same receiver settings to enable a critical generalization test of the trained deep learning (DL) model. The authors suggest training the DL model with the Day1 dataset with recordings under varied Bluetooth and WiFi signal strengths followed by evaluating the generalization capability of the model with the Day2 dataset which is a challenging and different setup compared to Day1. This evaluation will validate the realistic deployment capability of the trained DL model. The dataset follows the SigMF specifications with certain field extensions to facilitate the fingerprinting application and include additional metadata fields. Each capture is of length 40 Mega Samples (MS) and is associated with a JSON metadata file.
RF-FINGERPRINT-BT-IOT
REAL-WORLD FREQUENCY HOPPING BLUETOOTH DATASET FROM IOT DEVICES FOR RF FINGERPRINTING
A real-world radio frequency (RF) fingerprinting dataset for commercial off-the-shelf (COTS) Bluetooth emitters under challenging testbed setups is presented in this dataset. The dataset includes emissions from 10 COTS IoT emitters (2 laptops and 8 commercial chips) that are captured with a National Instruments Ettus USRP X300 radio outfitted with a UBX160 daughterboard and a VERT2450 antenna. The receiver is tuned to record a 2 MHz bandwidth of the spectrum centered at the 2.414 GHz frequency. This is a first-of-its-kind dataset for fingerprinting Bluetooth emitters under challenging and diverse indoor laboratory setups. The dataset is split into two: Day1 and Day2 each of which is recorded in a different time frame, location, and testbed setup to enable critical generalization test of the trained deep learning (DL) model. The authors suggest training the DL model with the Day1 dataset which is a simpler setup with recordings under varied Bluetooth signal strengths followed by evaluating the generalization capability of the model with Day2 dataset which is a challenging and vastly different setup compared to Day1. This evaluation will validate the real-world deployment capability of the trained DL model. The dataset follows the SigMF specifications with certain field extensions to facilitate the fingerprinting application and include additional metadata fields. Each capture is of length 40 Mega Samples (MS) and is associated with a JSON metadata file.
MR-iNET Gym
Marconi-Rosenblatt Framework for Intelligent Networks (MR-iNet Gym) For Rapid Design and Implementation of Distributed Multi-agent Reinforcement Learning Solutions for Wireless Networks
The first ns3 module for CDMA packaged to be driven by a reinforcement learning (RL) framework.
RadarCommDataset
RadarCommDataset is a wireless signal dataset released for public use under the Creative Commons Attribution – NonCommercial – ShareAlike 4.0 (CC BY-NC-SA 4.0) License by MR Lab at ANDRO. The lack of existing multitask labelled datasets for machine learning for wireless communication is the prime motivation urging this release. RadarCommDataset is first of its kind multitask labelled dataset released to help research community to advance machine learning for wireless communication. The dataset contains radar and communication waveforms. This repository supplements helper scripts to visualize as well as extract the dataset. Please contact for any other license. Use the following button to go to the RadarCommDataset Github page.
Publications
Research
ANDRO applies fundamental research to develop new technologies as a solution in many application areas. Take a look at a selection of our research publications.